Research
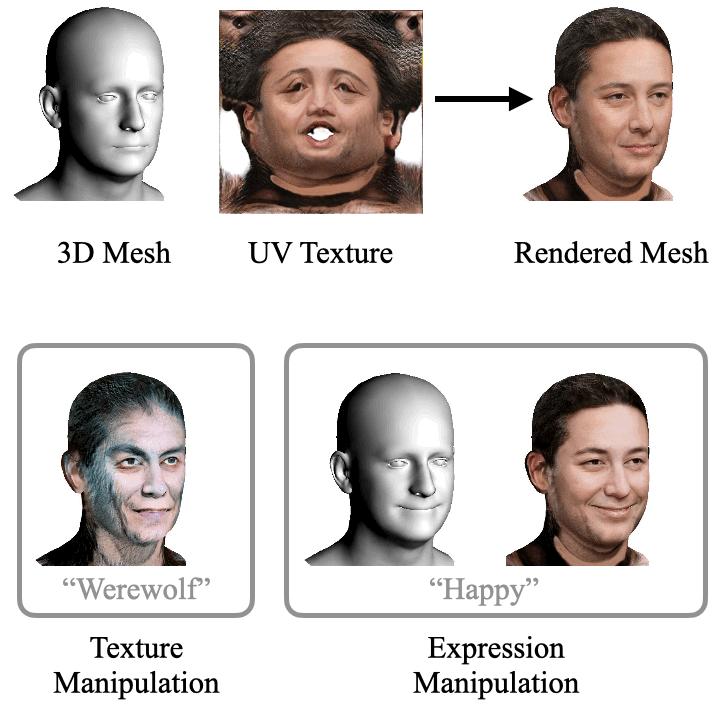
ClipFace learns a self-supervised generative model for jointly synthesizing geometry and texture leveraging 3D morphable face models, that can be guided by text prompts. For a given 3D mesh with fixed topology, we can generate arbitrary face textures as UV maps. The textured mesh can then be manipulated with text guidance to generate diverse set of textures and geometric expressions in 3D by altering (a) only the UV texture maps for Texture Manipulation and (b) both UV maps and mesh geometry for Expression Manipulation. Check out the paper for more details.
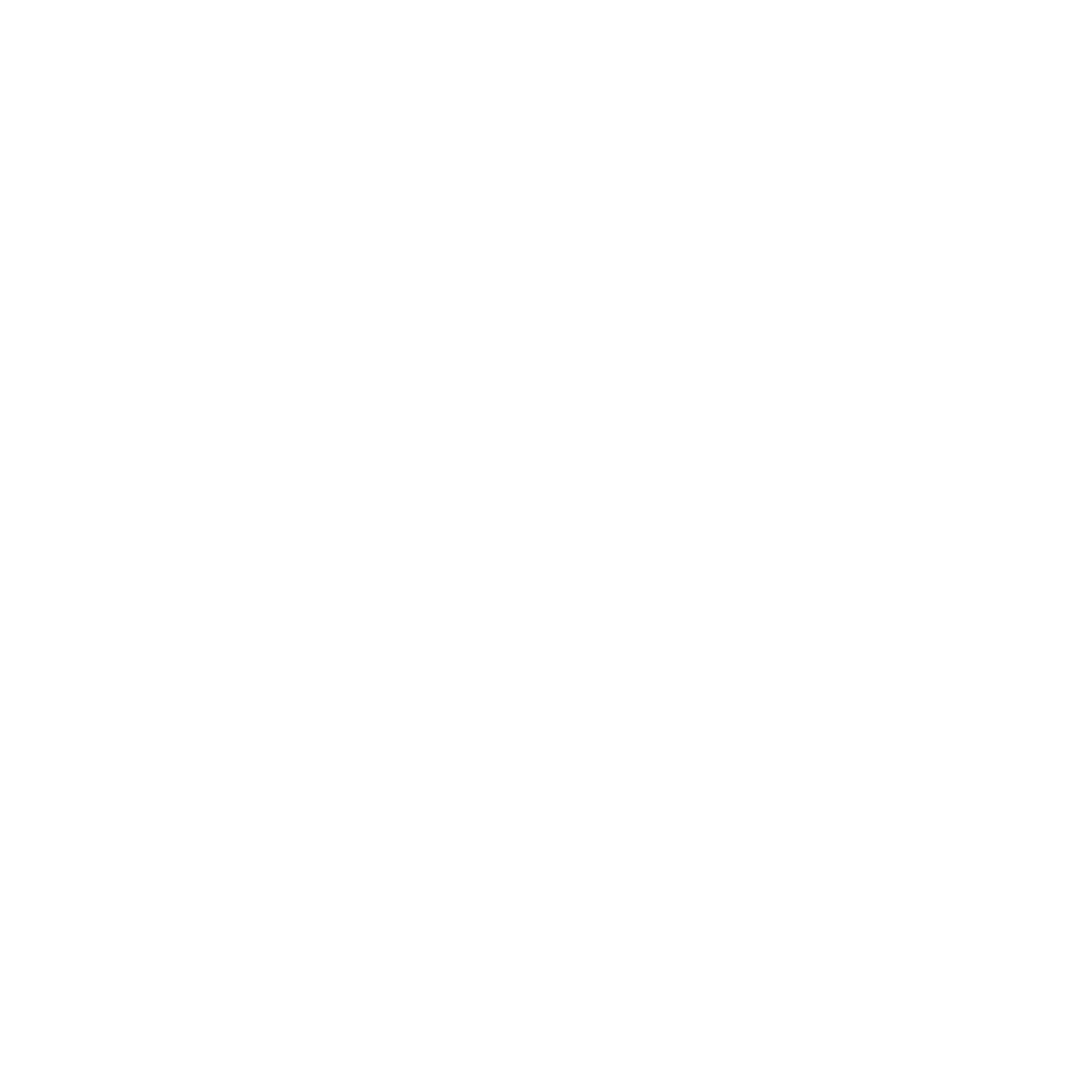
We introduce a novel data-driven approach that produces image-specific perturbations which are embedded in the original images to prevent face manipulation by causing the manipulation model to produce a predefined manipulation target. Compared to traditional adversarial attacks that optimize noise patterns for each image individually, our generalized model only needs a single forward pass, thus running orders of magnitude faster and allowing for easy integration in image processing stacks, even on resource-constrained devices like smartphones. Check out the paper for more details.
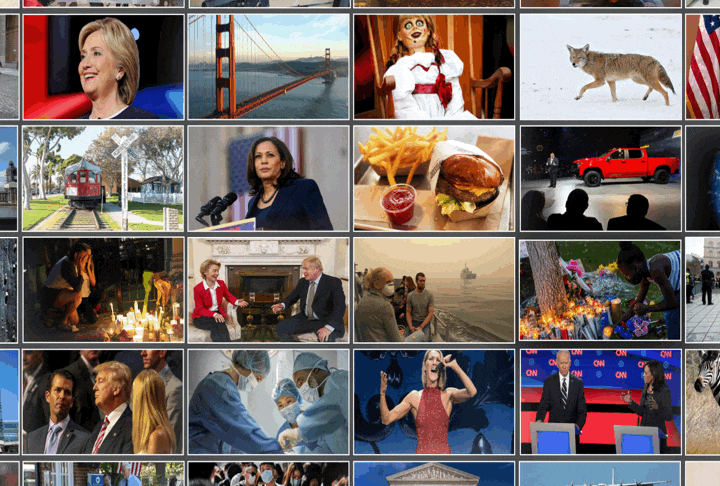
Despite the recent attention to DeepFakes, one of the most prevalent ways to mislead audiences on social media is the use of unaltered images in a new but false context. To address these challenges and support fact-checkers, we propose a new method that automatically detects out-of-context image and text pairs. Our key insight is to leverage grounding of image with text to distinguish out-of-context scenarios that cannot be disambiguated with language alone. Check out the paper for more details.
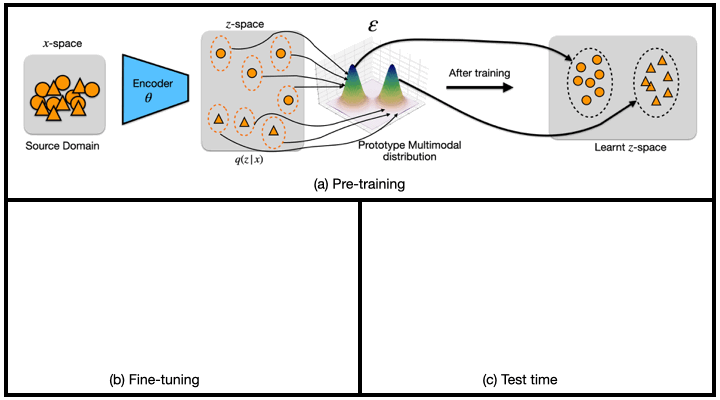
We propose a new transfer learning approach to address the problem of zero and few-shot transfer in the context of facial forgery detection. We examine how well a model (pre-)trained with one forgery creation method generalizes towards a previously unseen manipulation technique or different dataset. To facilitate transfer, we introduce a new mixture model-based loss formulation that learns a multi-modal distribution, with modes corresponding to class categories of the underlying data of the source forgery method. Check out the paper for more details.