Shivangi Aneja
I am a PhD candidate at Visual Computing and AI Lab at Technical University of Munich advised by Prof. Matthias Nießner. Prior to that, I obtained my Masters degree in Informatics from Technical University of Munich and Bachelors degree in Computer Science from National Institute of Technology, Hamirpur (India). My Master's thesis earned highest honors and was awarded the Best Master Thesis Award at DGOF Conference. During my undergrad, I was awarded a gold medal for academic excellence. My PhD research focuses on developing algorithms to generate lifelike and immersive 3D digital humans with expressive capabilities. Additionally, I also develop novel approaches that can thwart the malevolent usage of such generative models.
Publications
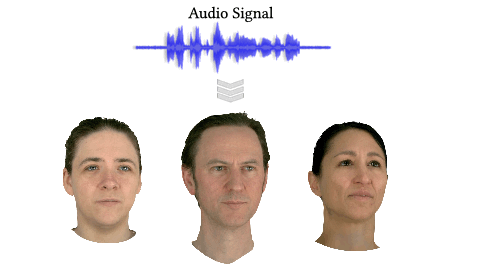
GaussianSpeech: Audio-Driven Gaussian Avatars
Shivangi Aneja, Artem Sevastopolsky, Tobias Kirschstein, Justus Thies, Angela Dai, Matthias Niessner
GaussianSpeech synthesizes high-fidelity animation sequences of photo-realistic, personalized 3D human head avatars from spoken audio. . Our method can generate realistic and high-quality animations, including mouth interiors such as teeth, wrinkles, and specularities in the eyes. We handle diverse facial geometry, including hair buns and mustaches/beards, while effectively generalizing to in-the-wild audio clips.
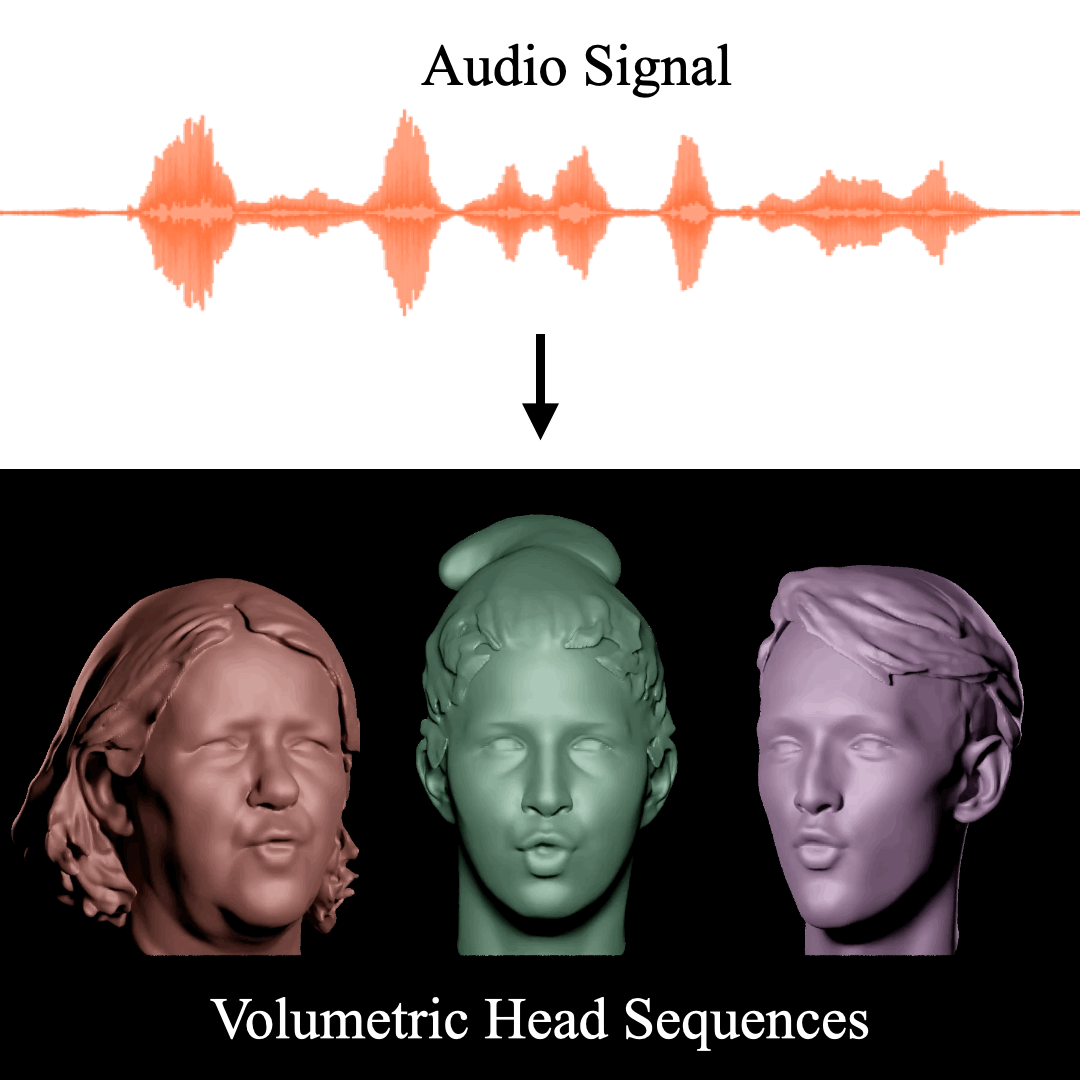
FaceTalk: Audio-Driven Motion Diffusion for Neural Parametric Head Models (CVPR 2024)
Shivangi Aneja, Justus Thies, Angela Dai, Matthias Niessner
Given input speech signal, FaceTalk can synthesize high-quality and temporally consistent 3D motion sequences of high-fidelity human heads as neural parametric head models. Our method can generate diverse set of expression sequences including foreign languages and songs. By optimizing for correspondences to produce temporally-optimized expressions fitted for audio supervision, we couple speech signal with latent space of neural parametric head model (NPHM) enabling coherent motion generation for arbitrary audios like songs and foreign languages.
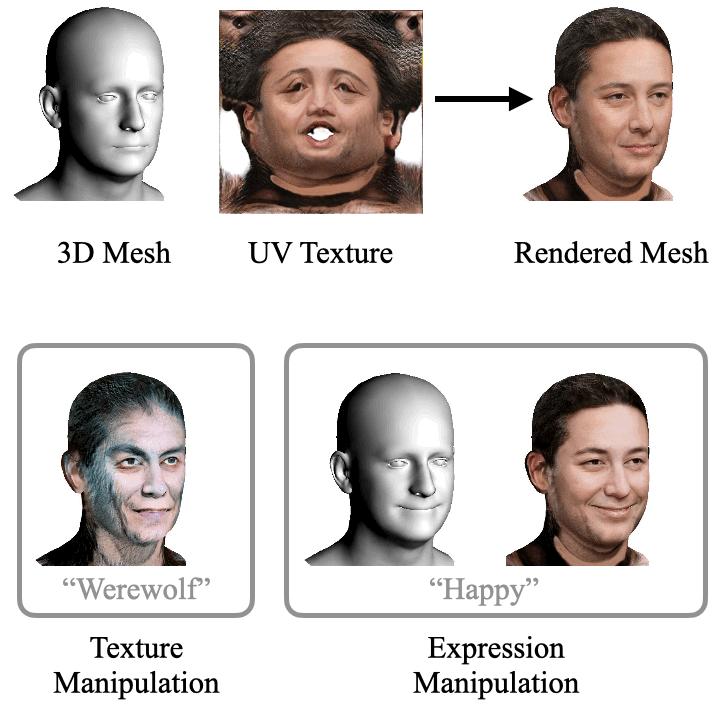
ClipFace: Text-guided Editing of Textured 3D Morphable Models (SIGGRAPH 2023)
Shivangi Aneja, Justus Thies, Angela Dai, Matthias Niessner
ClipFace learns a novel self-supervised approach for text-guided editing of textured 3D morphable model of faces. Specifically, we employ user-friendly language prompts to enable control of the expressions as well as appearance of 3D faces. We leverage the geometric expressiveness of 3D morphable models, which inherently possess limited controllability and texture expressivity, and develop a self-supervised generative model to jointly synthesize expressive, textured, and articulated faces in 3D. We enable high-quality texture generation for 3D faces by adversarial self-supervised training, guided by differentiable rendering against collections of real RGB images.
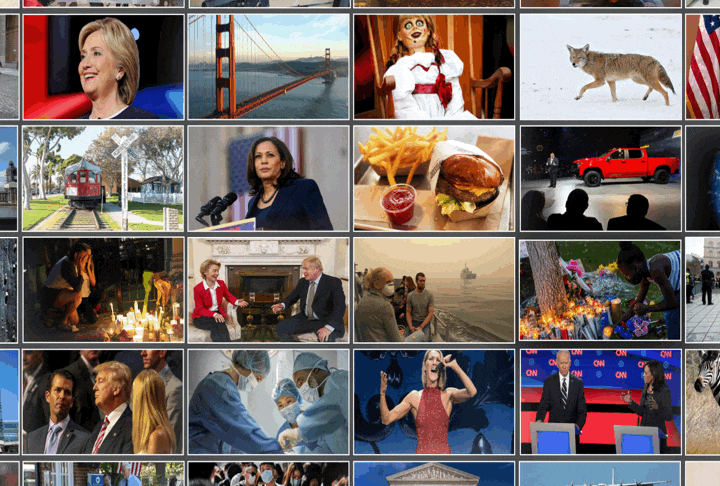
COSMOS: Catching Out-of-Context Misinformation using Self-Supervised Learning (AAAI 2023)
Shivangi Aneja, Chris Bregler, Matthias Niessner
One of the most prevalent ways to mislead audiences on social media is the use of unaltered images in a new but false context. To address this challenge and support fact-checkers, we propose a new method that automatically detects out-of-context image and text pairs. Our method takes as input an image and two captions from different sources, and we predict whether the image has been used out of context or not. We show that it is critical to the task to ground the captions w.r.t. image, and it is insufficient to consider only the captions; e.g., a language-only model would incorrectly classify the right image to be out of context.
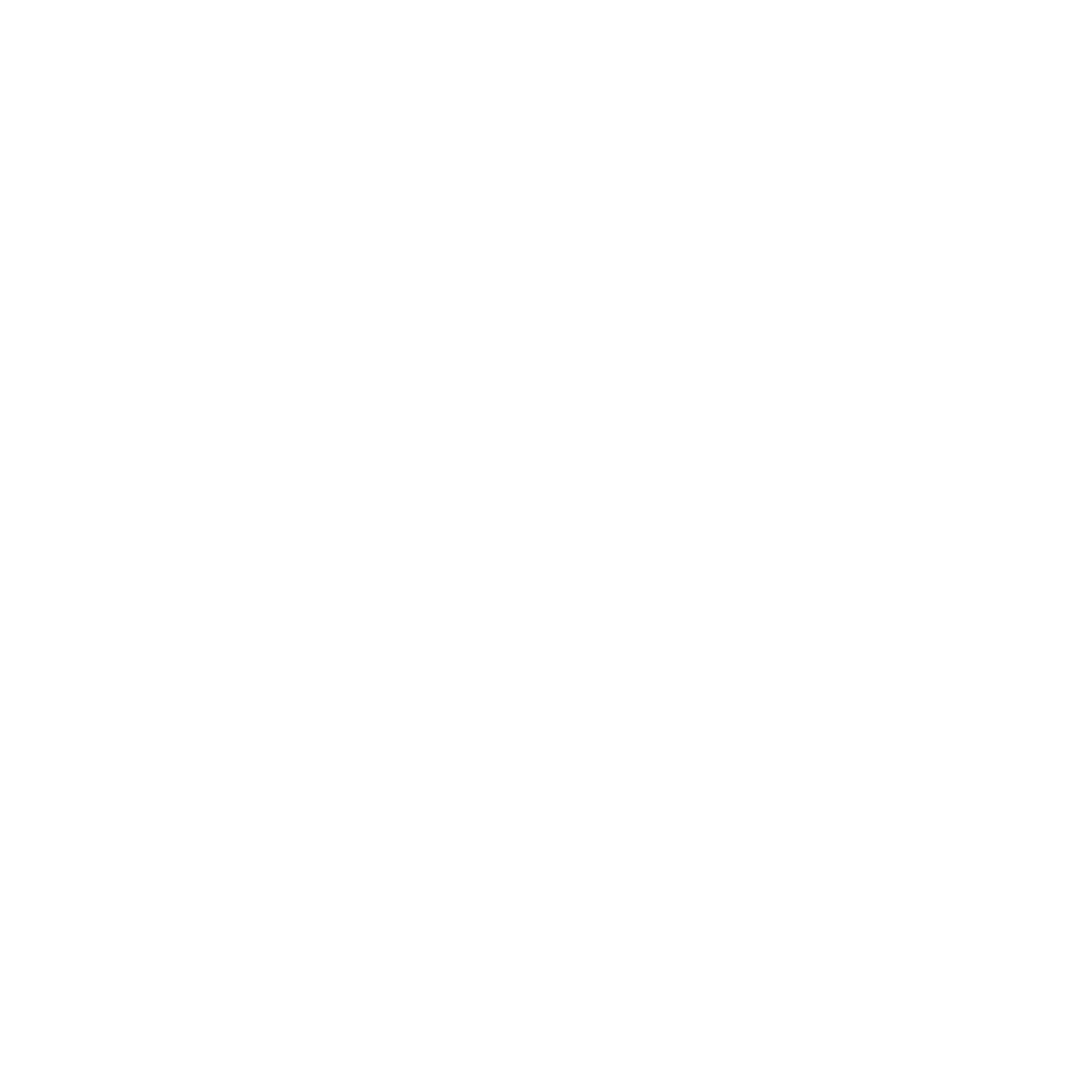
TAFIM: Targeted Adversarial Attacks against Facial Image Manipulations (ECCV 2022)
Shivangi Aneja, Lev Markhasin, Matthias Niessner
We propose a novel approach to protect facial images from several image manipulation models simultaneously. Our method works by generating quasi-imperceptible perturbations using a learned neural network. These perturbations when added to real images force the face manipulation models to produce a predefined manipulation target as output. Compared to existing methods that require an image-specific optimization, we propose to leverage a neural network to encode the generation of image specific perturbations, which is several orders of magnitude faster and can be used for real-time applications. In addition, our generated perturbations are robust to jpeg compression.
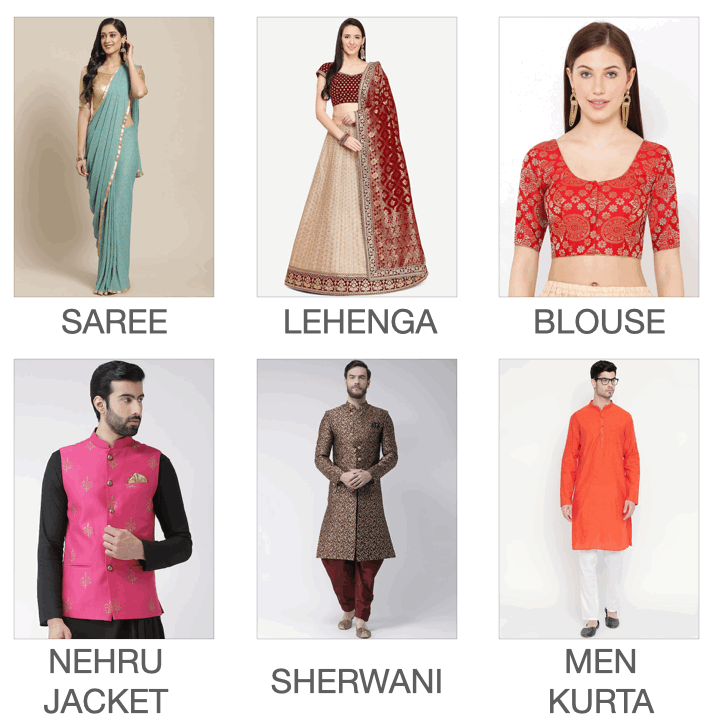
IndoFashion: Apparel Classification for Indian Ethnic Clothes (CVPRW 2021)
Pranjal Singh Rajput, Shivangi Aneja
Cloth categorization is used by e-commerce websites for displaying correct products to the end-users. Indian clothes have a large number of clothing categories both for men and women. Moreover, the style and patterns of ethnic clothes have a very different distribution from western outfits. Thus the models trained on standard cloth datasets fail on ethnic outfits. We introduce the first large-scale ethnic dataset of over 106K images with 15 different categories for fine-grained classification of Indian ethnic clothes. We evaluate several baselines for the cloth classification task on our dataset and obtain 88.43% accuracy.
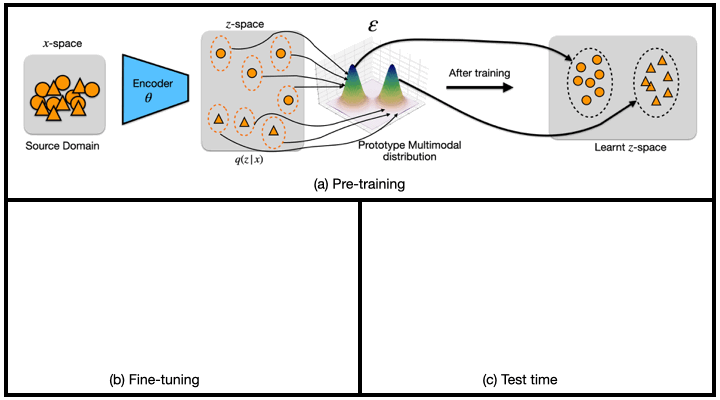
Generalized Zero and Few-Shot Transfer for Facial Forgery Detection (Master Thesis)
Shivangi Aneja, Matthias Niessner
We propose a new transfer learning approach to address the problem of zero and few-shot transfer in the context of facial forgery detection. We examine how well a model (pre-)trained with one forgery creation method generalizes towards a previously unseen manipulation technique or different dataset. To facilitate this transfer, we introduce a new mixture model-based loss formulation that learns a multi-modal distribution, with modes corresponding to class categories of the underlying data of the source forgery method. Our core idea is to first pre-train an encoder neural network, which maps each mode of this distribution to the respective class labels, i.e., real or fake images in the source domain by minimizing wasserstein distance between them. In order to transfer this model to a new domain, we associate a few target samples with one of the previously trained modes.